How the FDA
regulates medical devices
An introduction on medical device classification, regulation, and how to get a new product to market in the U.S.
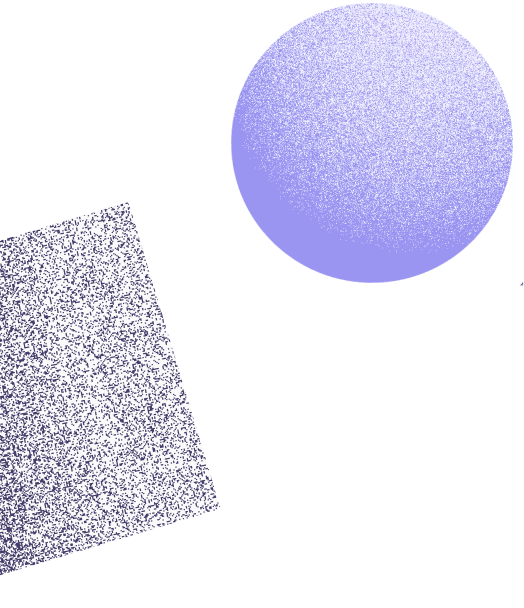
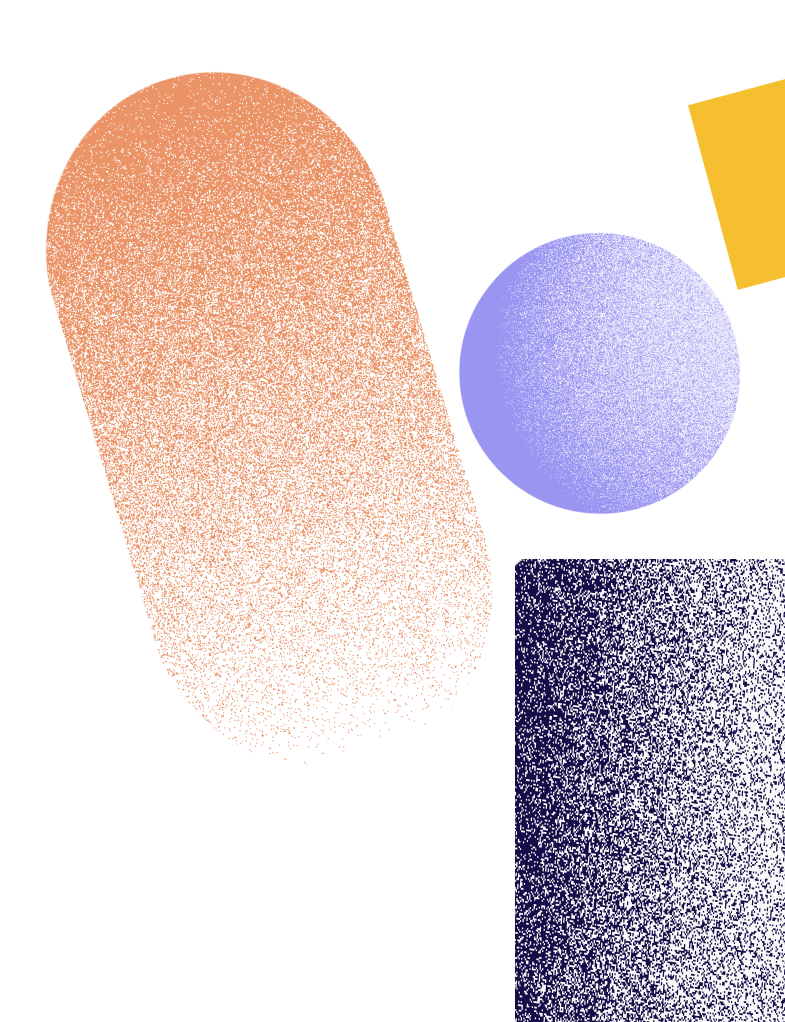
Is my product a medical device?
Examples of general controls
(21 Cfr part)
(21 Cfr part)
(21 Cfr part)
(21 Cfr part)
(21 Cfr part)
(21 Cfr part)
(21 Cfr part)
How to get a new product to market
step 1
Establish the Product
Identify product (device) description
Identify purpose
- intended use (usually broad)
- indications for use (more specific)
- duration of use
- target patient population (age range; disease)
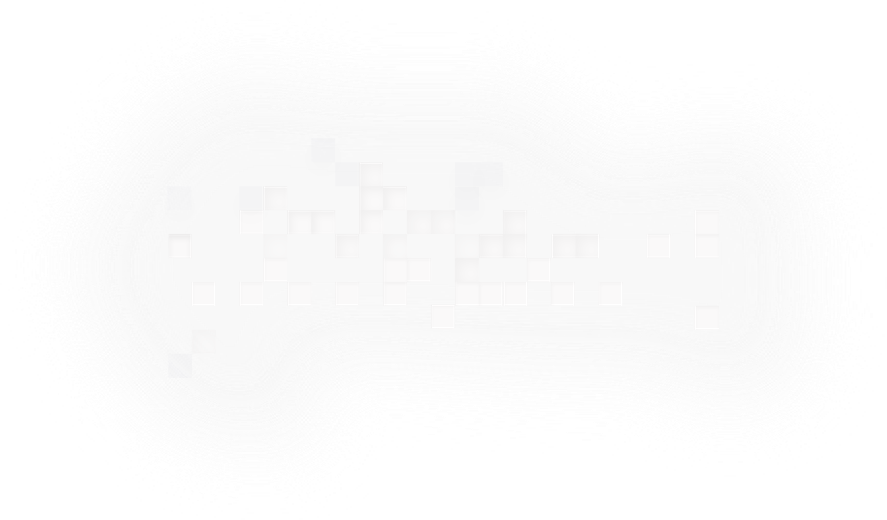
step 2
Verify the product is a medical device
Understand the FDA’s definition of medical device
Reference Section 201(h) of the Food, Drug & Cosmetic Act
step 3
Identify Classification and Regulatory Pathway
Identify regulatory classification
- Informal FDA opinion - email device determination office. devicedetermination@fda.hhs.gov
- Formal FDA opinion - file 513(g)
Classification will generally indicate regulatory pathway (premarket submission type) required for device
step 4
Develop valid scientific evidence
21 CFR 860.7(c)(1)
- Requires valid scientific evidence for safety and effectiveness
21 CFR 860.7(c)(2)
- Provides definition of valid scientific evidence
step 5
Prepare Premarket Submission
Each type has own sets of:
- processes
- applicable laws and regulations
- review times
- evidence burden
Establish the product
Identify product (device) description
Identify purpose
- intended use (usually broad)
- indications for use (more specific)
- duration of use
- target patient population (age range; disease)
Verify the product is a medical device
Understand the FDA’s definition of medical device
Reference Section 201(h) of the Food, Drug & Cosmetic Act
Identify classification and regulatory pathway
Identify regulatory classification
- Informal FDA opinion - email device determination office. devicedetermination@fda.hhs.gov
- Formal FDA opinion - file 513(g)
Classification will generally indicate regulatory pathway (premarket submission type) required for device
Develop valid scientific evidence
21 CFR 860.7(c)(1)
- Requires valid scientific evidence for safety and effectiveness
21 CFR 860.7(c)(2)
- Provides definition of valid scientific evidence
Prepare pre-market submission
Each type has own sets of:
- processes
- applicable laws and regulations
- review times
- evidence burden
Types of pre-market submissions
Investigational Device Exemption (IDE)
Clinical research on high-risk investigational devices
Collect safety and effectiveness data for future marketing application
Requires approval by Institutional Review Board
Intended to protect human patients
Premarket Notification 510(k)
Market application for low and moderate risk devices
“Substantial Equivalence” between new device and a legally marketed device
90-day review time
Premarket Approval Application (PMA)
Market application for highest risk devices
Reasonable assurance: safety and effectiveness
Evidence stands on own – not equivalence
180 - 320 day review
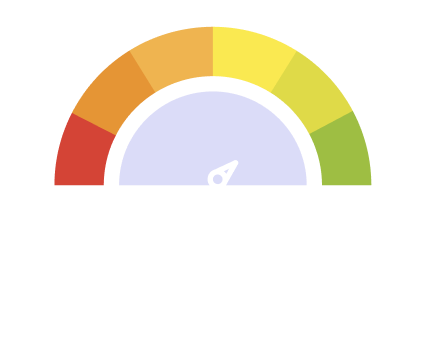
De Novo
Device has no existing classification regulation
Marketing process for novel devices
Creates new classification regulation
Alternative to PMA
Reduced regulatory burden/controls based on risk-benefit profile of device
Humanitarian Device Exemption HDE
Premarket submission for Humanitarian Use Devices
8000 individuals per year in United States
Exempt from effectiveness
Reasonable assurance of safety and probable benefit
Free FDA resources
Educational articles, videos, and more covering medical device regulation, guidance, and laws.
Written content
Hundreds of pages of total product lifecycle regulatory information
Over 30 regulatory categories
“How to” guides
Comprehensive regulatory education
Presentations, computer-based training, webinars
Over 100 modules
Most are less than 20 minutes
Mobile-friendly
Phone: (800) 638-2041
Hours of operation: 9 am - 12:30 pm; 1-4:30 pm ET
Email: dice@fda.hhs.gov
DICE will respond within 2 business days
The Division of Industry and Consumer Education (DICE) answers questions from the medical device industry.
Q-Submission Program
The Q-submission Program provides a mechanism to request interactions with the FDA related to medical device submissions.
Pre-Submission
Meeting
Pre-Submission Written Feedback
Specific questions
Recommend 3-4 substantial topics
Help guide product development, develop protocols, prepare premarket applications
AI/ML regulations
There are over 700+ AI/ML products cleared by the FDA. Many of them related to imaging.
FDA recognizes the benefits of AI/ML and is working to provide clear guidance and standard methods to regulate such products.
FDA requires following Design Controls which means every new release should go through V&V. For example, AI/ML can not be updated or released without proper Design Controls. In some cases a premarket notification may be needed.
A submission may contain an outline of possible algorithm changes which can be performed by the manufacturer without requiring FDA review. Referred to as Pre-determined Change Control Plan (or PCCP), see here
Guiding principles for AI/ML regulations
Multi-disciplinary expertise is leveraged throughout the total product life cycle
In-depth understanding of a model’s intended integration into clinical workflow, and the desired benefits and associated patient risks, can help ensure that ML-enabled medical devices are safe and effective and address clinically meaningful needs over the lifecycle of the device.
Good software engineering and security practices are implemented
Model design is implemented with attention to the “fundamentals”: good software engineering practices, data quality assurance, data management, and robust cybersecurity practices. These practices include methodical risk management and design process that can appropriately capture and communicate design, implementation, and risk management decisions and rationale, as well as ensure data authenticity and integrity.
Clinical study participants and data sets are representative of the intended patient population
Data collection protocols should ensure that the relevant characteristics of the intended patient population (for example, in terms of age, gender, sex, race, and ethnicity), use, and measurement inputs are sufficiently represented in a sample of adequate size in the clinical study and training and test datasets, so that results can be reasonably generalized to the population of interest.
Training data sets are independent of test sets
Training and test datasets are selected and maintained to be appropriately independent of one another. All potential sources of dependence, including patient, data acquisition, and site factors, are considered and addressed to assure independence.
Selected reference datasets are based upon best available methods
Accepted, best available methods for developing a reference dataset ensure that clinically relevant data are collected and the limitations of the reference are understood. Accepted reference datasets in model development and testing that promote and demonstrate model robustness and generalizability across the intended patient population are used.
Model design is tailored to the available data and reflects the intended use of the device
Model design is suited to the available data and supports the active mitigation of known risks, like overfitting, performance degradation, and security risks. The clinical benefits and risks related to the product are well understood, used to derive clinically meaningful performance goals for testing, and support that the product can safely and effectively achieve its intended use.
Focus is placed on the performance of the human-AI team
Where the model has a “human in the loop,” human factors considerations and the human interpretability of the model outputs are addressed with emphasis on the performance of the human-AI team, rather than just the performance of the model in isolation.
Testing demonstrates device performance during clinically relevant conditions
Statistically sound test plans are developed and executed to generate clinically relevant device performance information independently of the training data set. Considerations include the intended patient population, important subgroups, clinical environment and use by the Human-AI team, measurement inputs, and potential confounding factors.
Users are provided clear, essential information
Users are provided ready access to clear, contextually relevant information that is appropriate for the intended audience (such as health care providers or patients) including: the product’s intended use and indications for use, characteristics of the data used to train and test the model, known limitations, user interface interpretation, and clinical workflow integration of the model.
Deployed models are monitored for performance and re-training risks are managed
Deployed models have the capability to be monitored in “real world” use with a focus on maintained or improved safety and performance. When models are periodically or continually trained after deployment, there are appropriate controls in place to manage risks of overfitting, unintended bias, or degradation of the model that may impact the safety and performance of the model.
Interested to learn more?
Solving incredibly challenging and nuanced problems is our thing. Tell us about your project – it can make all the difference.
SCHEDULE a call